The rise of new pharmaceuticals is driving a paradigm shift in drug development. One of the key challenges, especially with evolving regulations, is aseptic manufacturing—a crucial requirement for producing protein-based injectable drugs. Helbling’s experts specialize in developing sophisticated solutions that seamlessly combine regulatory compliance, quality, scalability, and efficiency. By leveraging the Quality by Design (QbD) approach and advanced automation powered by machine learning (ML), they are helping shape the future of pharmaceutical production.
Advanced (bio-)pharmaceuticals as well as cell and gene therapy offer new ways to treat pathologies in areas such as cancer or autoimmune diseases. This is driving a paradigm shift in drug development. Because terminal sterilization is not possible, these protein-based injectable drugs require aseptic manufacturing. For the design and commissioning of aseptic production facilities, new regulations, such as the EU GMP Annex 1 for the Manufacture of Sterile Medicinal Products, provide guidance. However, for many manufacturers, this poses challenges that require new solutions.
Reducing human intervention as well as applying automation and digitalization are crucial for complying with the regulations. Isolators have become standard practice in the design of aseptic facilities. These rely on filtered air with unidirectional airflow in a sealed cabinet to provide a protective barrier around exposed materials. However, they require large investment and operational effort. To ensure Quality by Design (QbD), a holistic and systematic design approach includes technical and operational aspects, and provides a scale-up path from the early stage. It also saves costs and improves yield.
New opportunities for the design and operation of aseptic lines are also offered by new technologies such as machine learning (ML). While the potential of ML to improve pharmaceutical manufacturing is huge, its use in highly regulated environments requires considerable know-how for its validation.
Helbling has expertise in developing complex equipment and automation solutions, with a focus on challenging requirements in regulated industries such as pharma. Its experience includes viability analysis of client-specific aseptic lines, conceptual design of potential solutions, the efficient execution of technical projects, and commissioning. The systematic approach applied in the design of automation solutions is inherently in line with QbD. Current projects have a particular relevance to QbD.
The following points discuss ML applications for automating operations, robotics and automated processes in isolators, and a transient CFD simulation with a Helbling case study to reduce Time-to-Market of automated aseptic cells.
1. Application of Machine Learning (ML) for automating operations
There are benefits to using ML in aseptic manufacturing: established use cases that provide value include ML-based work item identification, robot grasp point generation and the quality control of work steps by a human or robotic arm. ML also opens up specific use cases inside an isolator, such as automated visual inspection of defects in primary containers during the fill & finish process. It can also detect robotic motions breaching the First Air principle.
As well as solving technical challenges when developing an AI/ML model, there are regulatory requirements to meet in a Good Manufacturing Practice (GMP) environment. Currently, there are no specific regulatory requirements on the use of AI in pharmaceutical production. However, regulatory frameworks including the EU GMP Annex 11 on Computerized Systems and GAMP5 provide general guidance. For example, the use of computerized systems should result in consistent product quality, process control, quality assurance and a stable risk profile across the process.
It follows that the process of developing and validating an AI/ML model requires a systematic approach for its successful application in a controlled manufacturing environment. It is essential to have a validated model for decision-making autonomy, as well as adaptability and adequate quality control measures. To ensure successful deployment, an end-to-end process must consider the complete lifecycle of an AI/ML model and its validation. These three points are particularly relevant:
- Lifecycle from concept to development and operation/monitoring (see Fig. 1)
- Deployment with level of control and autonomy (see factbox 1 & table 1 on the maturity model)
- Required validation measures
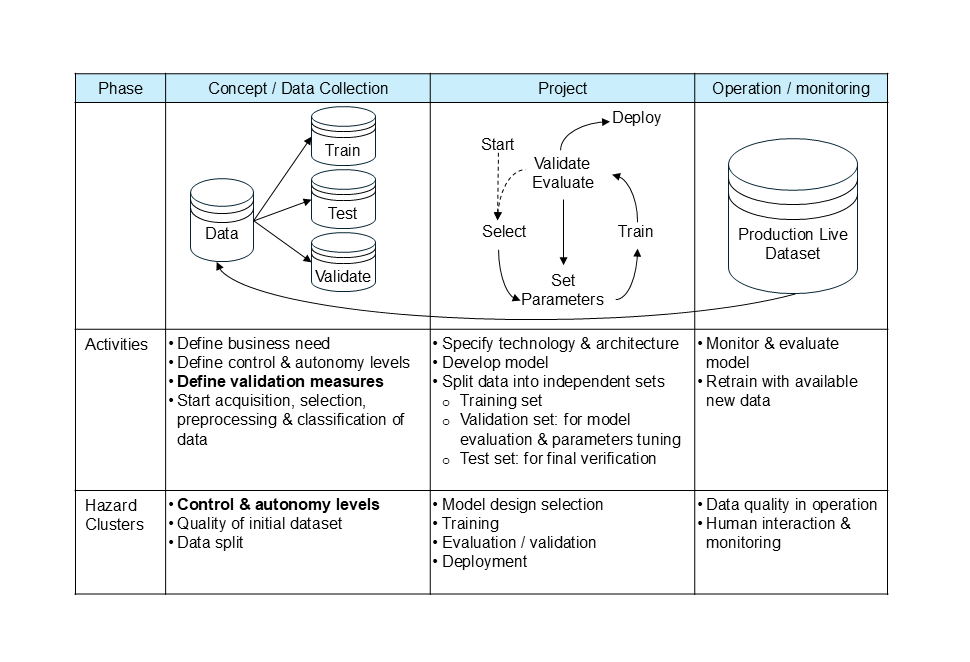
Application of Machine Learning
In the context of aseptic production, a practical application could include an automated visual inspection (AVI) quality control task, such as identification of cracks and particles (see Fig. 2). Different levels of control and autonomy are possible here.
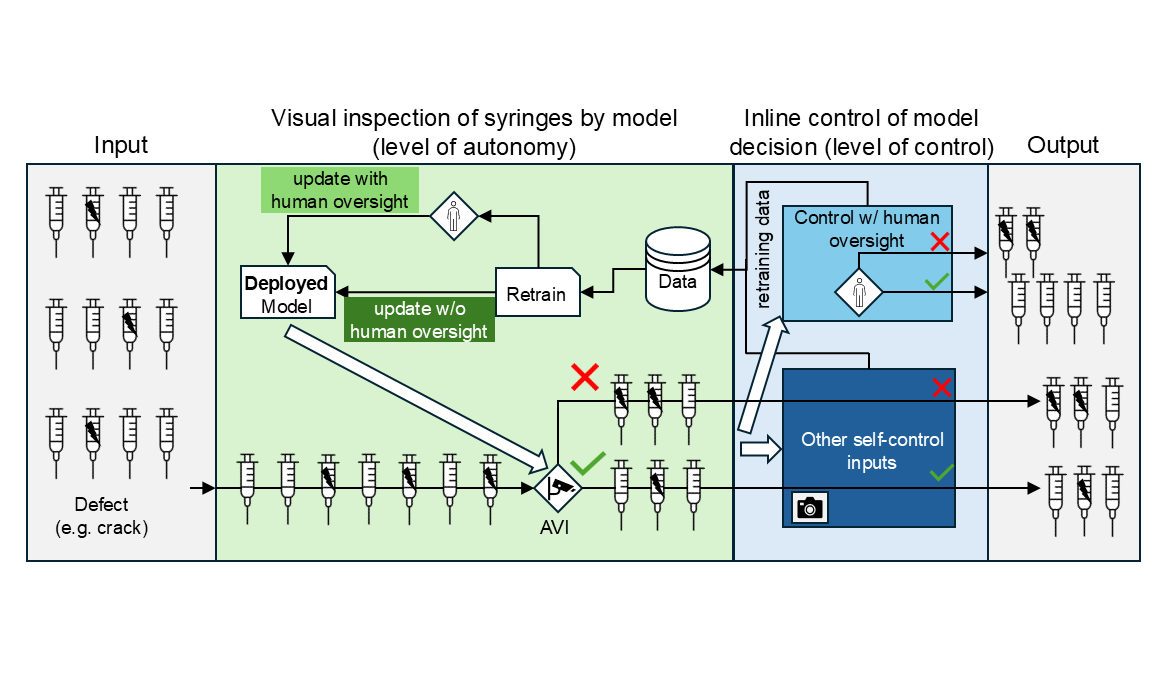
For a better overview of the relationship between the level of control and model autonomy, the following table summarizes the maturity model with validation levels. It also outlines the support Helbling can offer.
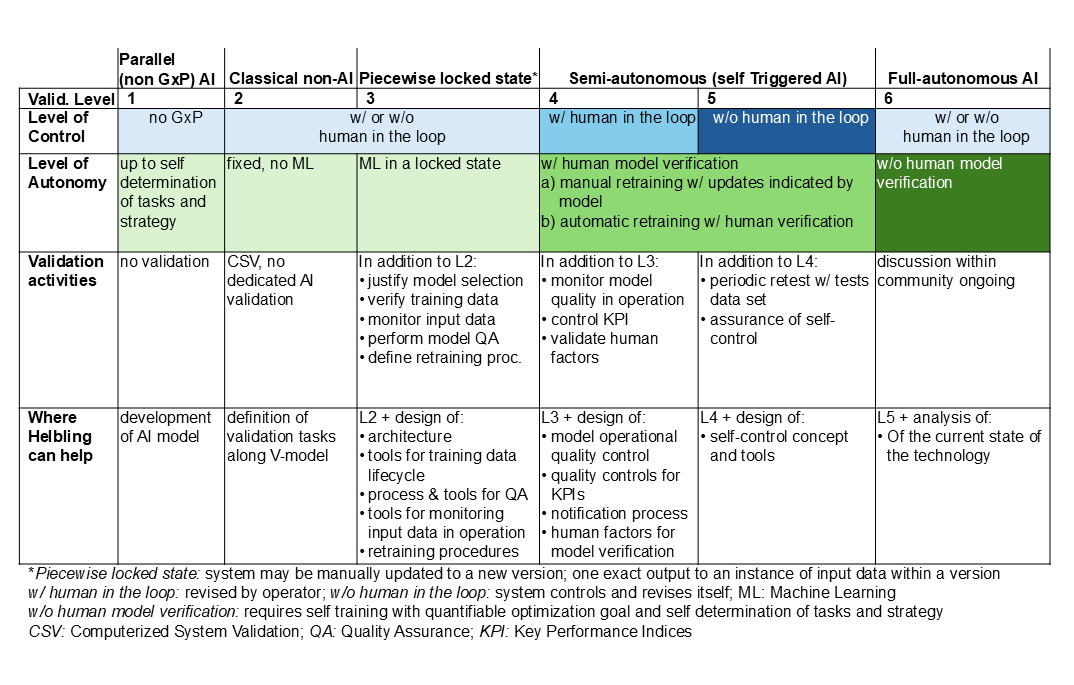
Helbling has experience in implementing AI and ML solutions in many industries, including the conception and acquisition of real-world or synthetic data, identifying the model architecture, and validation activities. With early-stage applications, regulatory agencies are open to discussion and Helbling can support the client’s dialogue with the authorities. This is crucial for the application of AI/ML in a regulated pharmaceutical environment.
2. Robotics and automated processes in isolatorsIn manually operated isolators, each step is carried out by trained personnel operating through hermetically sealed gloves so the throughput of a manual process is limited. There is also a higher risk of contamination due to variability in operator movement, touching product contact surfaces with gloves, and human error. Automation is equally becoming increasingly important for small-batch production of high-value drugs as it supports higher throughput, repeatability, and process traceability.
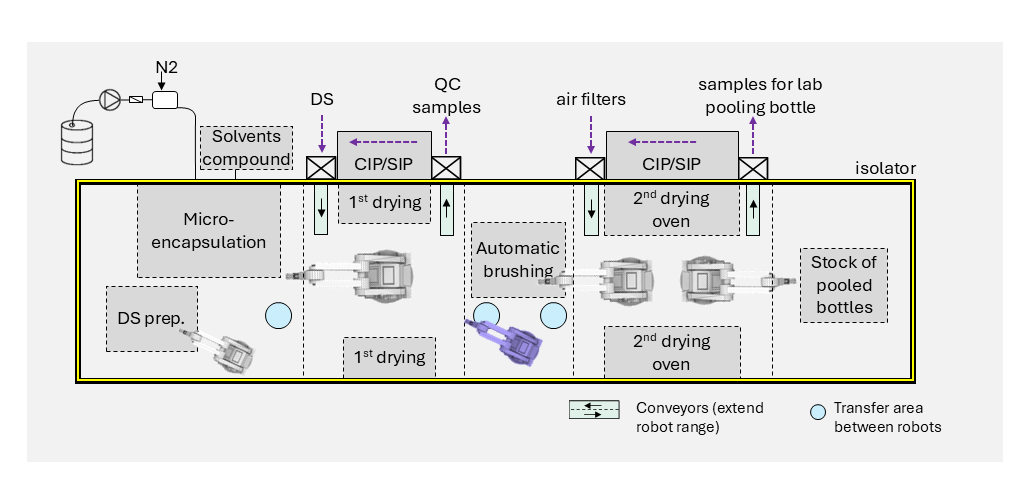
For small batches, there is a preference for robotic arms because they meet process quality requirements while offering flexibility for different primary packaging formats. Points to consider when choosing a robotic solution include:
- Tradeoff between speed and flexibility of 6-axes robots compared to classical XY stages
- Payload handling
- Compatibility with cleaning and sterilization processes (e.g. vaporized H2O2)
- Sealed design to minimize particle generation through wear
- Design of trajectory to minimize disturbance of First Air
The drug development and manufacturing process is long and complex. Drugs must go through lab, clinical, and pilot phases before finally reaching commercial scale. The must also avoid manufacturing process changes along the way. As a result, including scalability and modularity aspects from the start provides huge benefits that can generate tremendous time-to-market acceleration and cost savings.
3. Case Study: A Dynamic CFD Approach for Robotic Arm Motion in First Air
In a case study, Helbling examined an automated aseptic filling process with a six-axis robot arm in an isolator. It also looked at the interaction between air flow, cleanroom equipment, and the speed and acceleration of the robot’s movement. These robotic arms have a hygienic design that complies with GMP Grade A and ISO 14644-1 Class 5 cleanroom standards.
The principles of protective airflow are crucial to avoid contamination during the fill-and finish-step. Annex 1 introduces First Air management as a critical aspect. It shows that continuous, unidirectional and laminar sterile airflow must be ensured over the critical zones.
When it comes to new systems or new conditions in the environment, air flow patterns for sterile core zones must be visualized and validated as part of smoke studies. Because this is time-consuming, the aim is to ensure error-free production right from the start.
The case study used digital twins as tools for virtual development and commissioning as well as models in the field of computational fluid mechanics (CFD). Digital twins help to clarify air flow dynamics and optimize isolator design before any qualification studies in the real world. A holistic full-stack approach combines CFD with robot movement planning.
Findings from the case study
The case study proves the strength of this approach for the design and optimization of complex robotic aseptic applications. Digital twins can be leveraged to enhance process efficiency, safety, and compliance.
Integrating different software tools into this process involves combining heterogeneous, non-trivial interfaces. The procedure is iterative, encompassing motion planning, dynamic CFD simulation with components in motion, and trajectory optimization. Dynamic CFD can capture phenomena such as vortices and turbulence, which may not be revealed if motion is not accounted for.
The optimization process comprises several steps, starting with motion planning using general or supplier-specific tools. Next, it must be exported into a format suitable for dynamic CFD analysis. The latter includes mesh generation, boundary conditions and motion definition, computation of resulting airflows, and flow patterns visualization. Finally, robot motion and design (e.g gripper) must be optimized to ensure compliance.
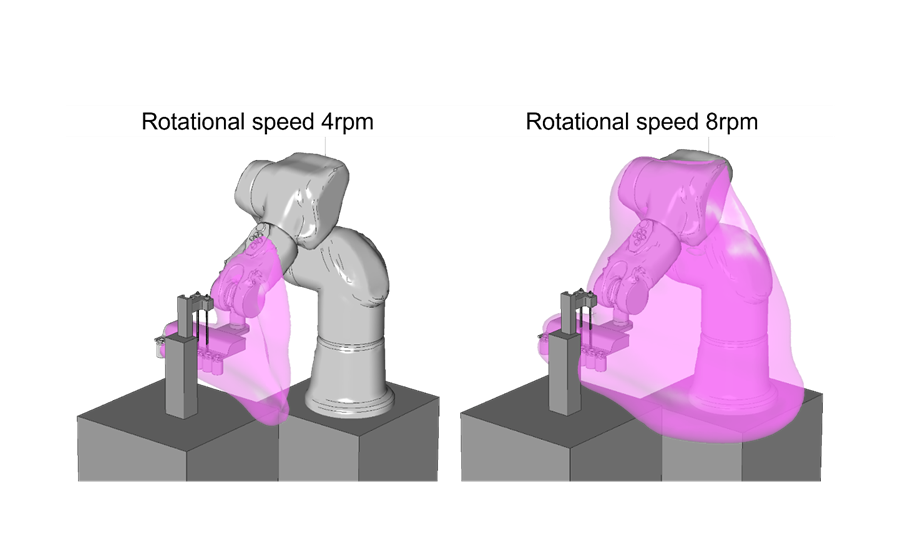
The case study identified the following:
- The tool helps find the optimum robot trajectory as the motion strongly influences streamlines and generates turbulent kinetic energy
- It helps optimize the equipment shape and aerodynamics as airflow accelerates and potentially recirculates when it flows over sharp edges
- Sterile air diffuser inlet must cover the entire critical working area to prevent eddies generation
Helbling specialist know-how in the industrial application of CFD simulations is key for setting up models at the sweet spot between accuracy and computation time, and to create a powerful optimization tool.
Summary: QbD and automation are crucial for the efficient production of new drugs
New regulations and advances in therapeutic forms are making the advantages of machine learning and automation solutions increasingly relevant for pharmaceutical companies and their partners. Here, Helbling can contribute the optimum range of experience. A systematic quality-by-design approach allows added value created by AI/ML models to unfold in regulated industries. With automation, it is important to maintain focus throughout the process to reconcile benefits and costs. Finally, dynamic CFD simulations help to integrate moving robot arms into production, shortening time-to-market and reducing costs.
Authors: Alex Rüegg, René Hartnack, Simon Dössegger, Franck Robin
Main Image: Dall-e
[1] ISPE: AI Maturity Model for GxP Application: A Foundation for AI Validation, Nico Erdmann, Rolf Blumenthal, Ingo Baumann, Markus Kaufmann https://ispe.org/pharmaceutical-engineering/march-april-2022/ai-maturity-model-gxp-application-foundation-ai
[2] ISPE: Machine Learning Risk and Control Framework, Rolf Blumenthal, Nico Erdmann, Martin Heitmann, Anna-Liisa Lemettinen, Brandi Stockton https://ispe.org/pharmaceutical-engineering/january-february-2024/machine-learning-risk-and-control-framework?utm_campaign=2024%20PE&utm_content=278828325&utm_medium=social&utm_source=linkedin&hss_channel=lcp-214189