Project
Root Cause Motor Failure Assessment
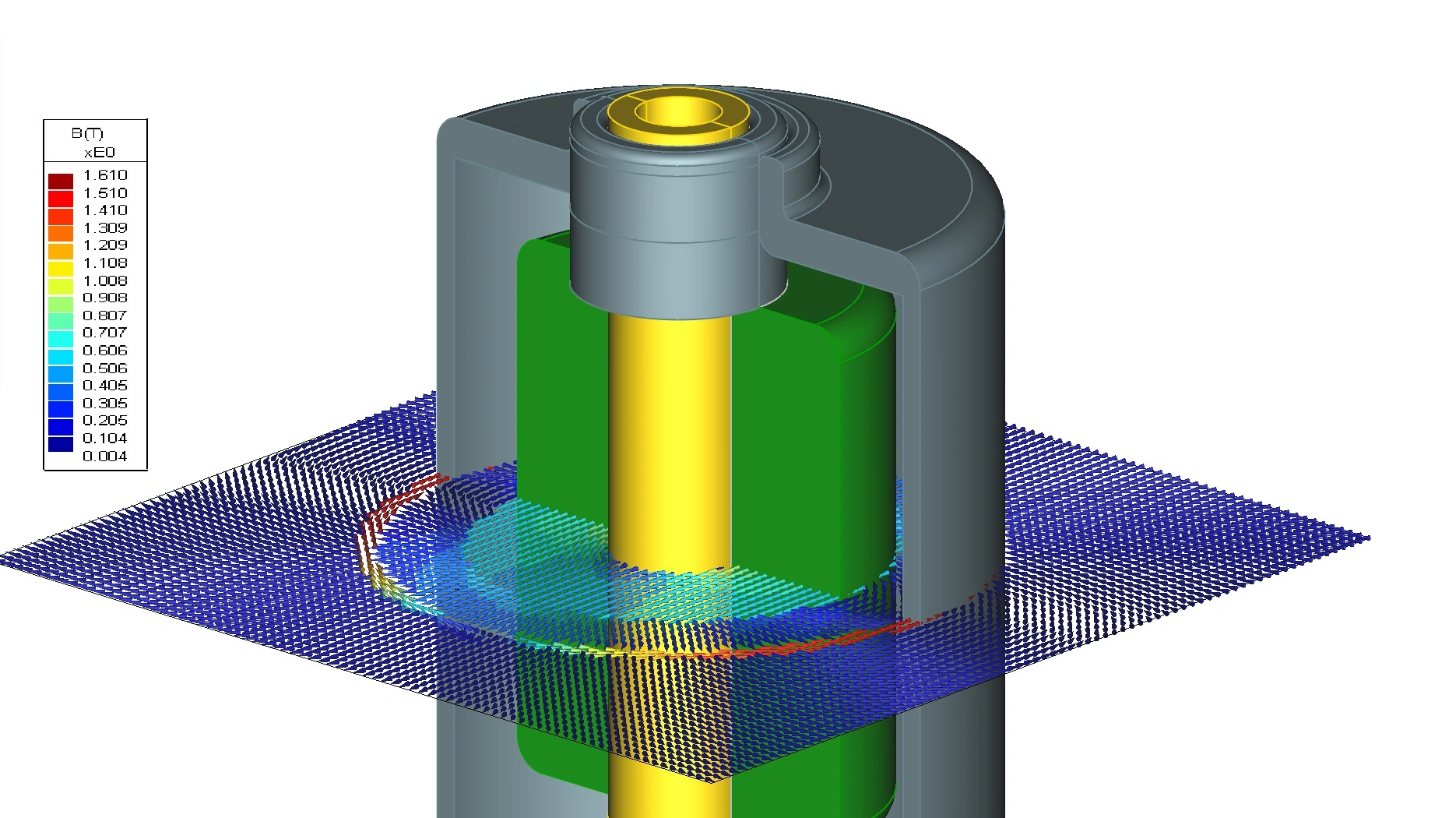
Our client was in the process of introducing a novel, wearable electromechanical drug delivery device to the market. As part of the production ramp-up efforts, our client observed several rare occurrences of motor start-up failures in fully assembled devices. The extreme rarity of incidence of the failure (<0.1%) implied that the root cause of the problem was elusive and difficult to find with only traditional, manufacturing-oriented forensic and inspection techniques. Our client complied a large set of motor torque data, which was the starting point for our project; to understand the root cause and provide measures to address them.
First, to gain an understanding of all possible sources of the motor failure, we built a theoretical model of the miniature motor and its supporting subsystems. The model formed the basis for a stochastic simulation, that was used as an analysis and prediction tool to determine the most influential parameters to the observed failure. Second, and complementary to our first approach, we used machine learning and neural network techniques to utilize the large torque data set and solve this non-linear problem. Combining the output of both approaches allowed us to develop measures to help our client.
First, to gain an understanding of all possible sources of the motor failure, we built a theoretical model of the miniature motor and its supporting subsystems. The model formed the basis for a stochastic simulation, that was used as an analysis and prediction tool to determine the most influential parameters to the observed failure. Second, and complementary to our first approach, we used machine learning and neural network techniques to utilize the large torque data set and solve this non-linear problem. Combining the output of both approaches allowed us to develop measures to help our client.
Key Figures
- Theoretical model of the generalized torque generated by the motor rotor
- Detailed 3D FEA of the magnetic field strength inside the motor
- Stochastic model of the variability of appropriate key parameters of the motor model
- Monte Carlo simulation predicting the variation of the startup torque
- Neural Network (NN) development using Bayesian Regularization
- Training of NN to improve its accuracy and categorize motors for potential malfunction
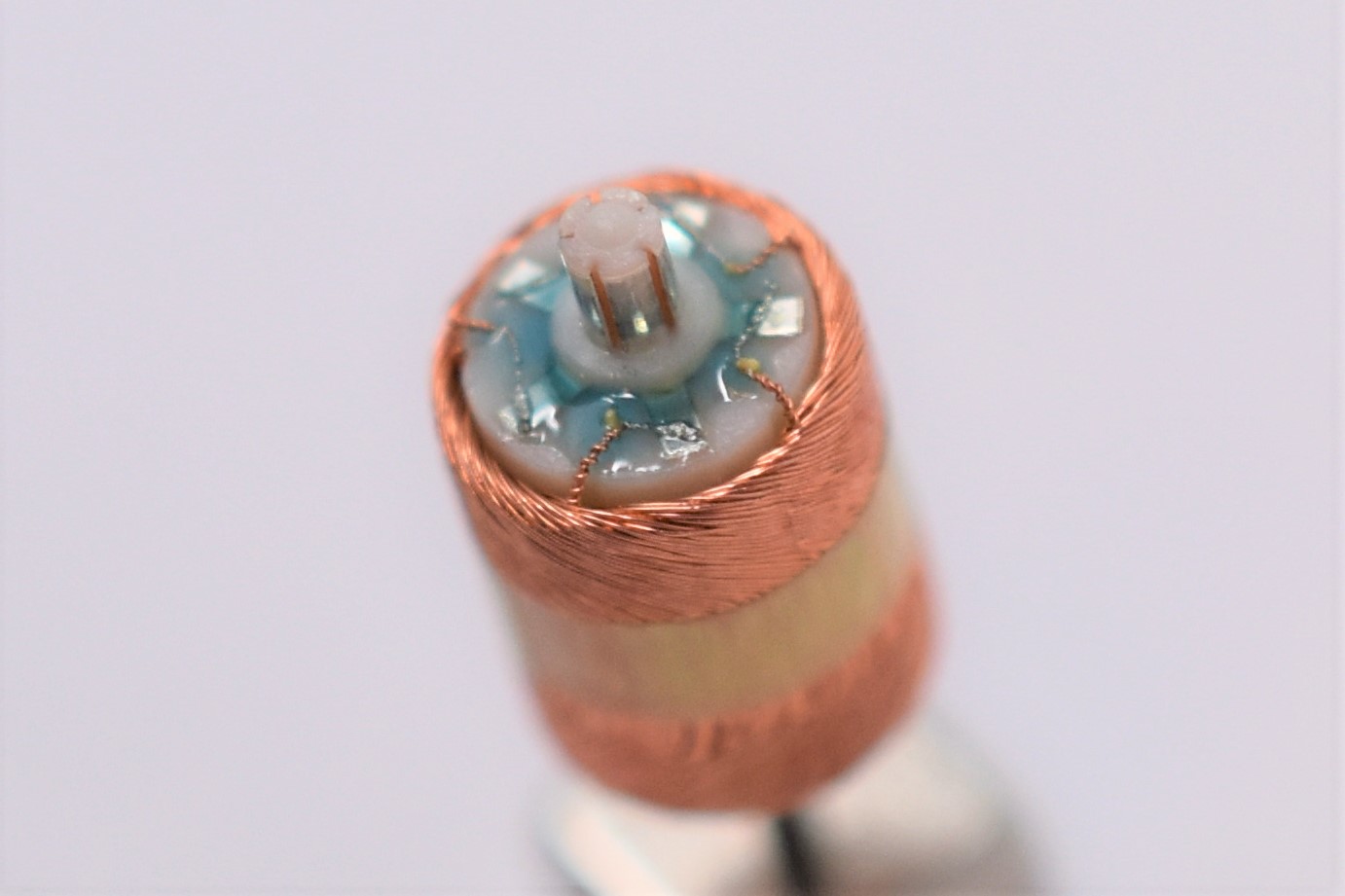
Our Contribution
- Performed detailed measurements and inspection of the provided motor samples to build an accurate motor CAD model
- Created detailed theoretical model of the motor startup condition and supporting systems
- Identified key parameters that affect motor startup performance
- Performed FEA analysis of motor’s magnetic fields
- Performed failure mode analysis and develop measures to address them
- Developed multi-variable and deep Neural Network based on client motor torque data to categorize motors
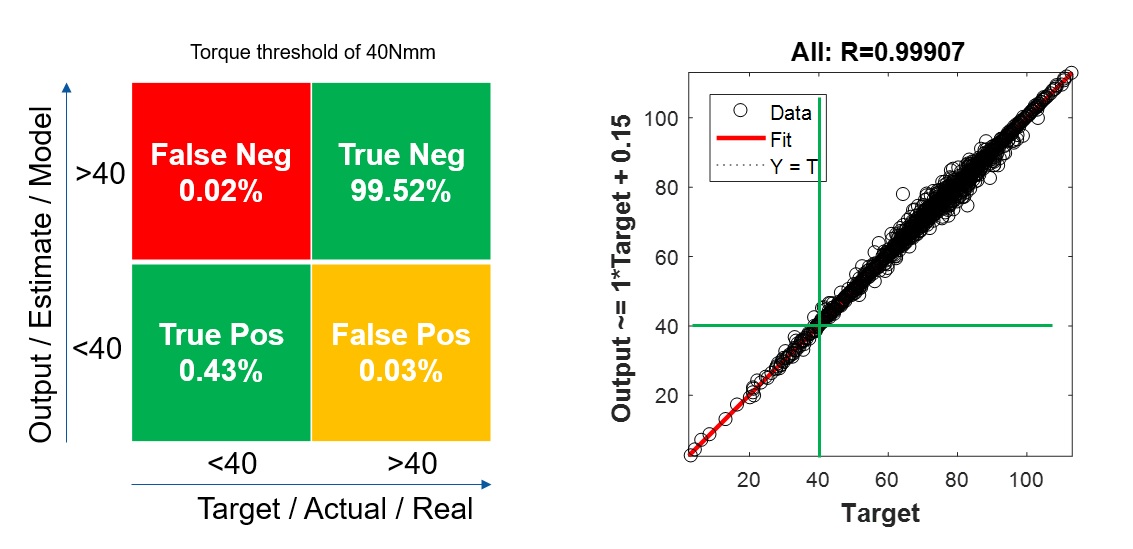
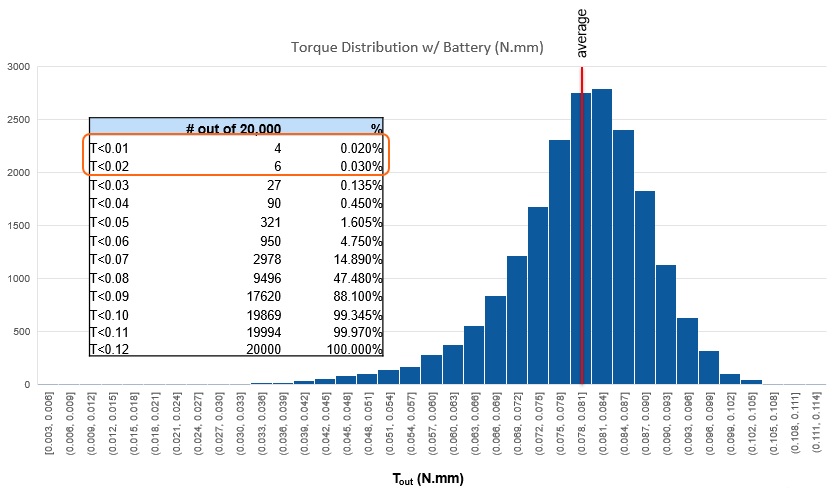
Outcome
By linking the traditional analysis model with a deep learning network, successful troubleshooting measures could be developed.
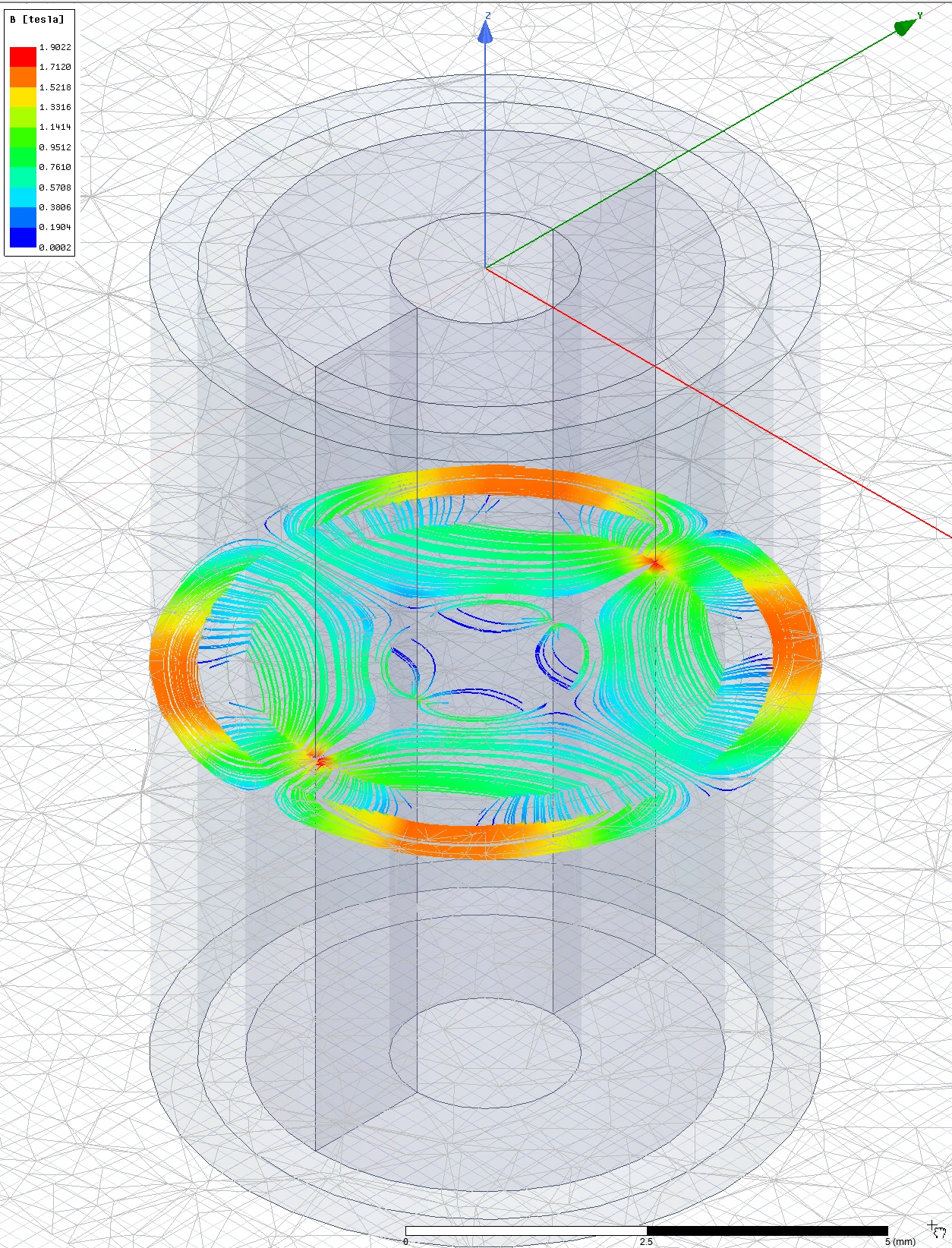
Contact
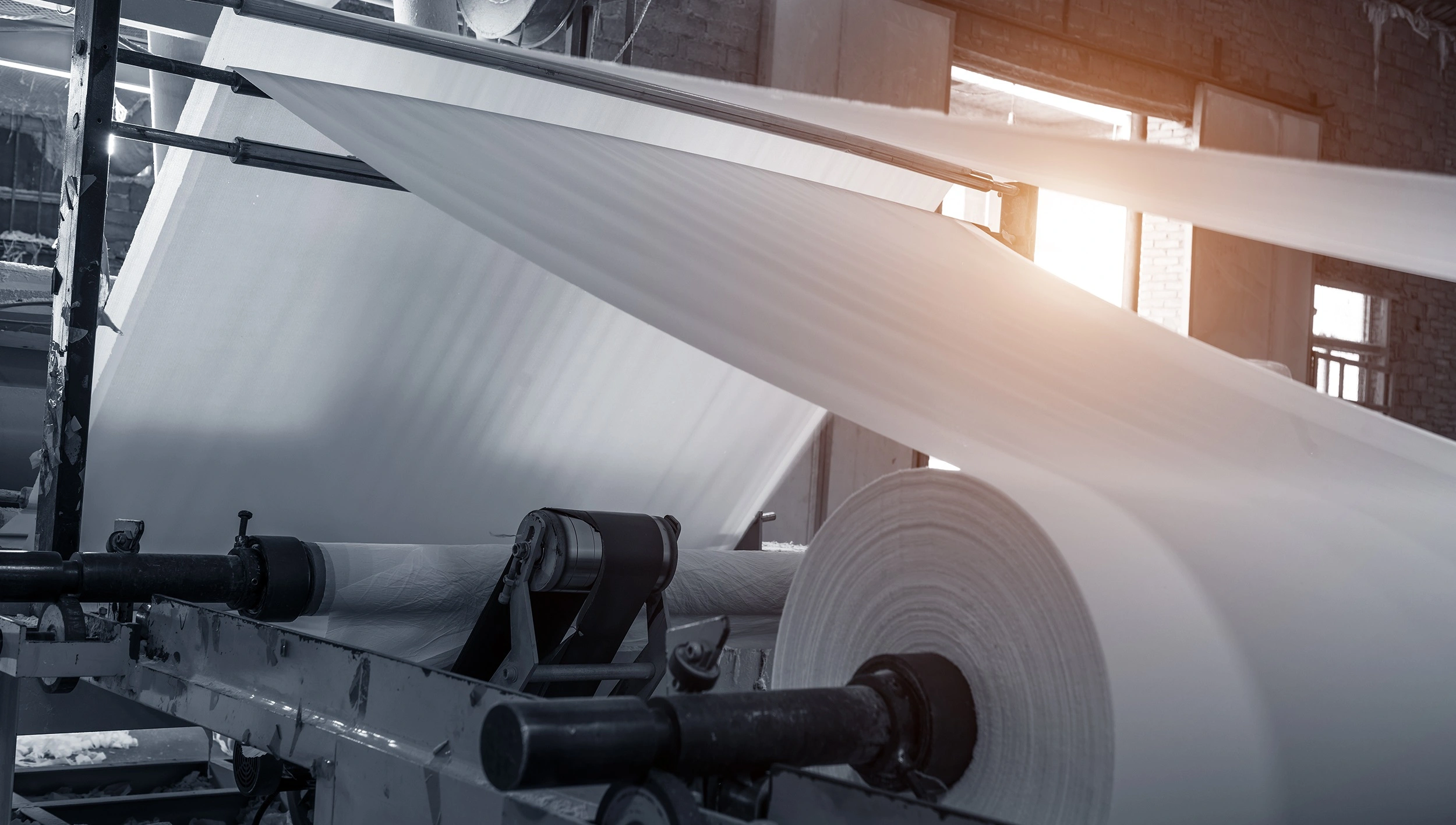
Cases
Internationales Unternehmen der Papierindustrie – Analyse des Dampfsystems
Industries:
Services:
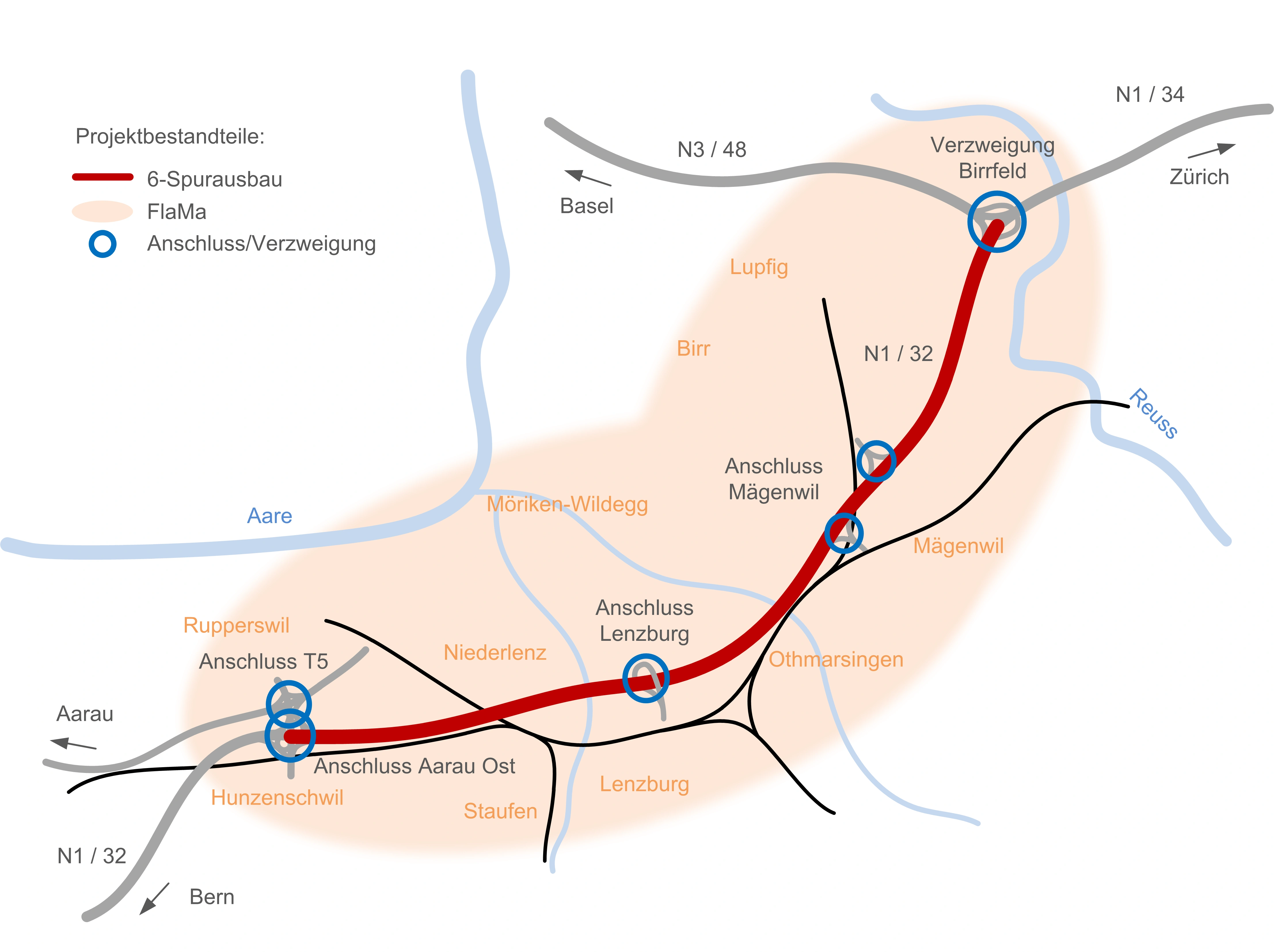
Cases
6-Streifenausbau Aarau Ost – Verzweigung Birrfeld – Bauherrenunterstützung
Industries:
Services: